With the digitization of health data and the application of machine learning and analytics, researchers, clinicians, and administrators are developing and adopting new tools to improve patient outcomes, reduce healthcare delivery costs and accelerate drug development pipelines.
However, lack of access to critical health data limits practitioners’ ability to unlock and drive impact with AI in healthcare.
The Impracticality of Data Centralization
Health data is generated across thousands of institutions and clinics across borders (and even within institutions), and is produced by a number of different devices, staff, and departments. Outside of healthcare, the primary approach to applying machine learning and analytics on distributed data is to attempt to break through data silos by centralizing the data in a data lake or data warehouse. However, three unique characteristics of health data have rendered centralization to be frequently impractical or even impossible: sensitivity, volume, and interoperability.
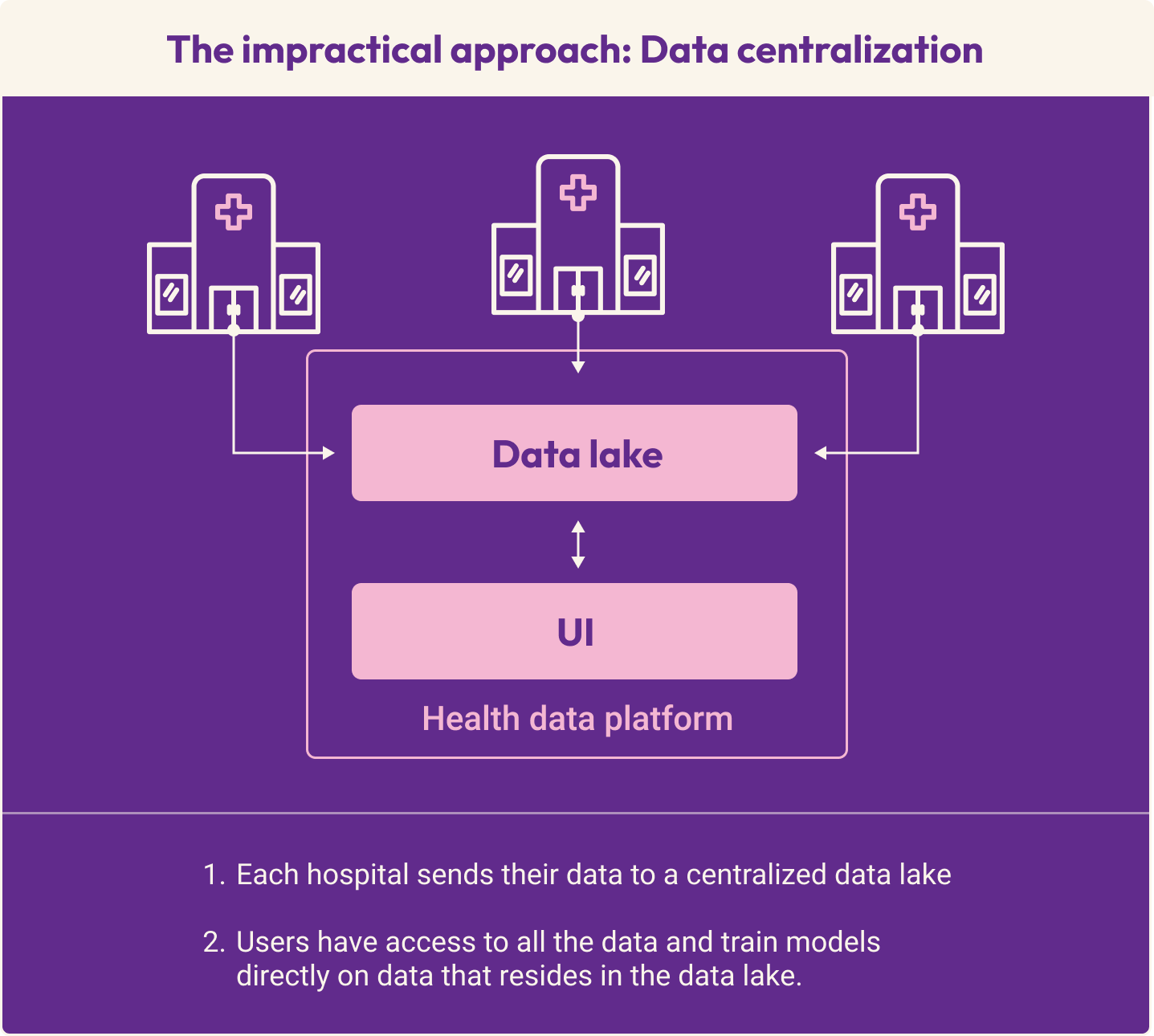
Sensitivity
Most countries have regulations limiting the usage of personal data, and many have supplemented their regulations with further guidance on protecting personal health information. GDPR in the EU and HIPAA in the U.S. severely limit the sharing of health data between institutions or across borders without express consent. Health data custodians also have their own privacy and security protocols, as well as concerns with sharing intellectual property which give them a competitive advantage.
Health institutions and product developers have traditionally managed these trust barriers through a combination of technical de-identification and legal means, but each has significant limitations. Because of the complexity and cost associated with sharing health data, many potentially high-value initiatives are slow or impossible to get off the ground — a major loss for researchers and patients.
Volume
The explosion of health data unlocks new opportunities for researchers to improve existing models with new features, and build new predictive models for diagnostics, precision medicine, and real-world evidence. But the promise of boundless health innovation driven by the sheer volume of digital health data must be tempered by the practical implications of moving and storing copies of these massive data sets.
The compute time and costs required to centralize data for machine learning and analytics severely restrict health AI innovation.
Interoperability
A historical lack of data standards in healthcare also creates challenges for data aggregation across sites. Hospital electronic health record (EHR) systems are designed to optimize hospital operations and comply with local rules and regulations, not to facilitate data sharing. Converting existing data to a standard format so that it can be aggregated across systems is both time-consuming and costly.
Efforts such as the Fast Healthcare Interoperability Resources® (FHIR®) open-source framework in the U.S. are underway to establish and enforce better health data standards. Challenges with adoption still exist outside the U.S., and interoperability does not solve the challenges of sharing sensitive data at scale.
The Limitations of Distributed Health Data
History has proven that barriers to sharing health data for machine learning and analytics hinder the overall progression of AI in healthcare. The cost and complexity of consolidating data make centralization impractical, while distributed systems place significant limitations on the ability to extract insights from siloed data. Simply put, the existing approaches to using machine learning and analytics on health data are no longer working and it is time for a new approach. It’s time to stop trying to break through data silos and, instead, focus on activating them.
Check out our blog continuation here to learn more about how researchers and data scientists can activate health data silos with federated learning.